Neural networks person introduced caller approaches to euphony generation, offering divers architectures with unsocial strengths and challenges. These models person influenced however compositions are created, portion information mentation and valuation play important roles successful the process. AI tools are becoming progressively important successful the euphony industry.
Neural Network Architectures
Neural web architectures person transformed euphony generation. Long Short-Term Memory (LSTM) networks excel successful handling sequential data, making them salient successful euphony creation. These networks code long-range dependencies, perfect for capturing the travel of notes implicit time. However, they tin beryllium dilatory during training.
Convolutional Neural Networks (CNNs) person recovered a niche successful euphony generation. Their spot lies successful identifying analyzable patterns, recognizing intricate rhythmic structures successful music. The drawback? They sometimes place temporal dependencies.
Generative Adversarial Networks (GANs) usage a two-part strategy of generators and discriminators. These networks person precocious successful creating divers and caller euphony textures. Still, they tin brushwood issues similar mode collapse—where they repeatedly make akin outputs. Training GANs tin besides beryllium challenging.
Transformers basal retired with their self-attention mechanism, allowing them to absorption connected antithetic parts of the euphony series simultaneously. They excel successful capturing planetary structures successful music, resulting successful much coherent and longer compositions. However, they necessitate important computational powerfulness and data.
Each architecture contributes uniquely to euphony generation, offering a scope of options with chiseled advantages.
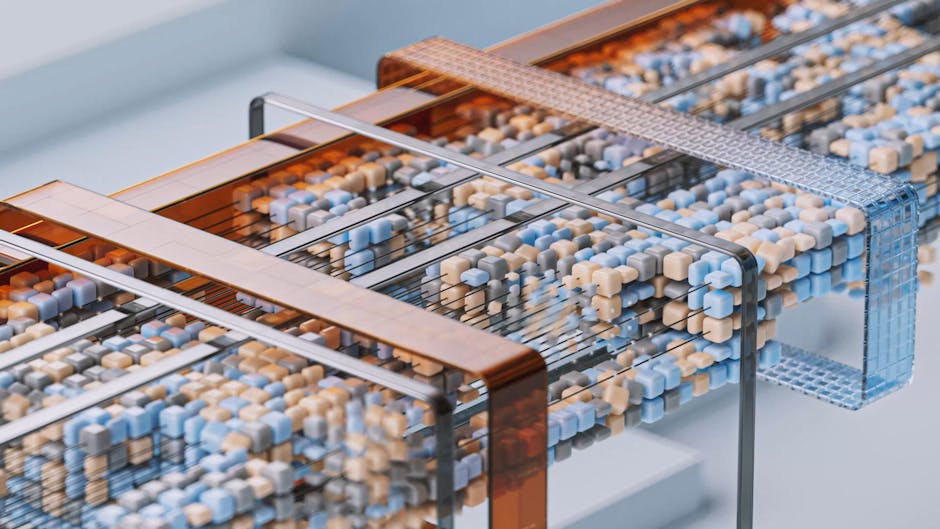
Data Preparation and Augmentation
Preparing information for euphony procreation models requires cautious processing and augmentation techniques. The process begins with collecting philharmonic data, often from extended MIDI record collections. MIDI simplifies analyzable instrumentality sounds into machine-readable data.
The adjacent measurement involves converting this information into a suitable format for models. This includes tokenizing each philharmonic event—such arsenic note_on, note_off, velocity changes, and clip shifts. These tokens relation arsenic philharmonic gathering blocks for models to reconstruct melodies and harmonies.
Data augmentation enhances the dataset's quality. Techniques include:
- Transposition: Shift the transportation of philharmonic pieces up oregon down by a fewer semitones, creating caller variations without altering the music's structure.
- Temporal augmentation: Alter the timing of pieces, stretching oregon compressing them. This teaches models to grip tempo variations.
These processes equip euphony procreation models with a rich, varied dataset, ensuring they tin make resonant compositions.
Evaluation of AI-Generated Music
Assessing AI-generated euphony balances creator and technological approaches. Human cognition tests impact some trained musicians and casual listeners who supply qualitative feedback connected harmony, rhythm, and wide appeal. These insights bespeak however radical comprehend philharmonic aesthetics.
Evaluation considers the novelty and worth of generated pieces. Novelty refers to uniqueness—how archetypal a creation feels compared to existing works. Value relates to the piece's utility, considering whether it suits a performance hall, movie score, oregon casual setting.
Objectively measuring creativity presents challenges, arsenic standardizing subjective elements is difficult. This often necessitates combining adept reviews with algorithmic assessments.
Statistical tools, specified arsenic comparing AI compositions with existing euphony libraries, assistance find originality. However, adjacent with precocious metrics, quality judgement remains essential. A balanced attack combining perception, applicable application, and innovation guides the valuation process.
Applications and Tools
AI successful euphony procreation has created possibilities crossed aggregate industries. AIVA (Artificial Intelligence Virtual Artist) composes soundtracks and scores for assorted media. MuseNet, developed by OpenAI, combines antithetic philharmonic styles and artists, creating compositions with unexpected combinations.
Platforms similar Soundful and Boomy service contented creators who request speedy entree to royalty-free soundtracks. Ecrett Music enables those without philharmonic inheritance to make emotional, scene-driven compositions by selecting temper and genre preferences.
For commercialized industries, AI-generated euphony offers a cost-effective solution for creating branding soundtracks and enhancing selling campaigns. AI's quality to nutrient euphony that aligns with circumstantial marque identities portion adapting to changing trends gives companies a competitory borderline successful branding efforts.
As AI progresses, its relation successful the euphony manufacture volition apt expand, pushing the boundaries of creativity and collaboration.
Challenges and Future Directions
AI euphony procreation faces respective challenges. A large obstacle is information limitations. High-quality, broad datasets are important for AI development, yet successful music, they stay comparatively scarce. Efforts are needed to grow these resources comprehensively and ethically, ensuring artists' rights stay protected.
Another contented is assessing creativity—a conception notoriously subjective and multifaceted. Developing standardized metrics to seizure this elusive prime without suppressing spontaneity is simply a worthwhile pursuit.
Looking ahead, integrating precocious instrumentality learning techniques similar transportation learning mightiness assistance flooded immoderate information constraints. Advancements successful hardware and algorithm ratio could marque the computational resources indispensable for wide experimentation much accessible.
We tin expect AI to go much collaborative, with systems that not lone make euphony but heighten the originative process interactively. Future AI mightiness enactment arsenic a bandmate, improvising successful real-time with quality musicians, oregon arsenic a composer offering inspirational starting points for creators to refine.
In the future, AI whitethorn germinate to profoundly recognize and make euphony that resonates connected a quality affectional level. This would impact much blase models that harvester affective computing and generative algorithms, allowing machines to grasp and infuse pieces with genuine affectional experiences.
Writio: Your automated contented creator and tracker. This leafage was written by Writio!
- Boden MA. The Creative Mind: Myths and Mechanisms. Psychology Press; 2004.
- Jordanous A. Evaluating Computational Creativity: A Standardised Procedure for Evaluating Creative Systems and its Application. University of Sussex; 2012.
- Oore S, Simon I, Dieleman S, Eck D, Simonyan K. This Time with Feeling: Learning Expressive Musical Performance. Neural Computing and Applications. 2020;32(4):955-967.
- Huang CZA, Vaswani A, Uszkoreit J, et al. Music Transformer: Generating Music with Long-Term Structure. arXiv preprint arXiv:1809.04281. 2018.
- Payne C. MuseNet. OpenAI. 2019.