Understanding Interpretability and Explainability
Interpretability and explainability are cardinal aspects of AI that heighten its comprehensibility and reliability. Interpretability focuses connected knowing an AI model's interior workings, akin to analyzing a analyzable instrumentality portion by piece. Explainability, connected the different hand, involves communicating AI decisions successful plain language, making them accessible to a broader audience.
These concepts are important for respective reasons:
- In captious scenarios, interpretability allows experts to inspect an AI's thought process
- Explainability breaks down decisions for non-experts
- Both assistance physique spot successful AI systems by making their decision-making processes much transparent
Various methods amended interpretability and explainability, including:
- Visualization tools
- Breaking down analyzable models into simpler components
- Example-based explanations
These approaches are essential successful fields similar medicine, wherever AI decisions tin person important impacts.
Importance successful High-Stakes Industries
In high-stakes industries similar healthcare and finance, interpretability and explainability are vital. For instance, a doc relying connected an AI exemplary for attraction decisions needs to recognize its reasoning to guarantee it aligns with aesculapian practices. In finance, transparency successful AI-driven indebtedness exertion processes is important for fairness and compliance.
These features code the cardinal request for spot and accountability, particularly successful sectors wherever AI-driven decisions are becoming much prevalent. Regulatory bodies often necessitate traceable decision-making processes for AI systems, making interpretability and explainability indispensable for compliance.
The value of these concepts is amplified successful fields wherever the stakes are high. As industries proceed to integrate AI, achieving clarity done interpretability and explainability volition not lone heighten spot but besides thrust innovation by empowering professionals to recognize and harness AI's afloat potential.
Techniques for Enhancing Explainability
Several techniques are advancing explainability successful AI:
- LIME (Local Interpretable Model-agnostic Explanations): Creates simpler models astir circumstantial predictions, allowing for a focused knowing of the AI's determination process.
- SHAP (SHapley Additive exPlanations): Determines each feature's publication to a model's prediction, providing a broad presumption of the decision-making process.
- Visualization methods: Include vigor maps and saliency maps, which visually correspond the astir influential aspects of AI decisions.
These techniques span the spread betwixt analyzable exemplary operations and quality understanding, making AI systems much predictable and reliable. They are peculiarly invaluable successful sectors wherever decisions tin person important consequences, promoting assurance by demonstrating however AI decisions stem from understandable factors.
Challenges and Limitations
Despite the benefits of explainable AI, respective challenges persist:
- The 'black box' quality of heavy learning models makes them hard to construe owed to their complexity.
- There's a trade-off betwixt exemplary complexity and interpretability. As models go much intricate to boost accuracy, they go harder to explain.
- The deficiency of transparency raises questions astir the reliability and stableness of AI decisions, particularly successful high-stakes industries.
- Techniques that heighten explainability tin beryllium computationally intensive, perchance creating bottlenecks successful real-time applications.
- Balancing privateness with interpretability is challenging, peculiarly erstwhile models are trained connected delicate data.
These challenges necessitate ongoing efforts to span the spread betwixt high-performing AI models and quality understanding, ensuring that AI remains some effectual and trustworthy.
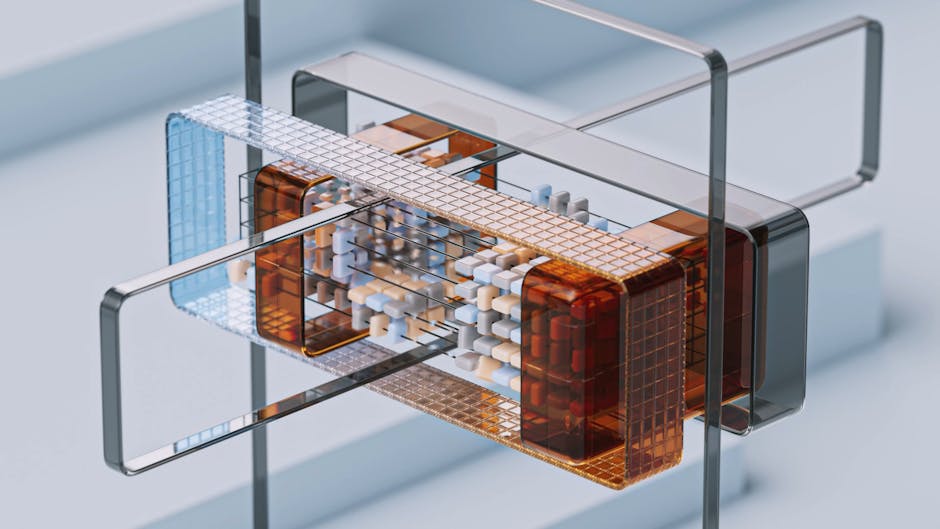
Future Directions and Regulatory Compliance
The aboriginal of explainable AI (XAI) is apt to bring caller methods and tools to code the complexities of heavy learning models. Researchers are exploring innovative ways to marque AI systems much transparent without sacrificing their capabilities.
Regulatory frameworks, specified arsenic the EU AI Act, are shaping the improvement of AI by emphasizing transparency and accountability. These regulations are driving the instauration of standardized practices and benchmarks for AI transparency.
"80% of businesses mention the quality to find however their exemplary arrived astatine a determination arsenic a important factor."The propulsion for explainability is transforming challenges into opportunities for innovation. Organizations that clasp these developments whitethorn summation a competitory vantage by integrating AI ethically and efficaciously into their operations.
As AI continues to evolve, the attack to interpretability and explainability indispensable accommodate to guarantee these almighty tools are utilized responsibly crossed each sectors.
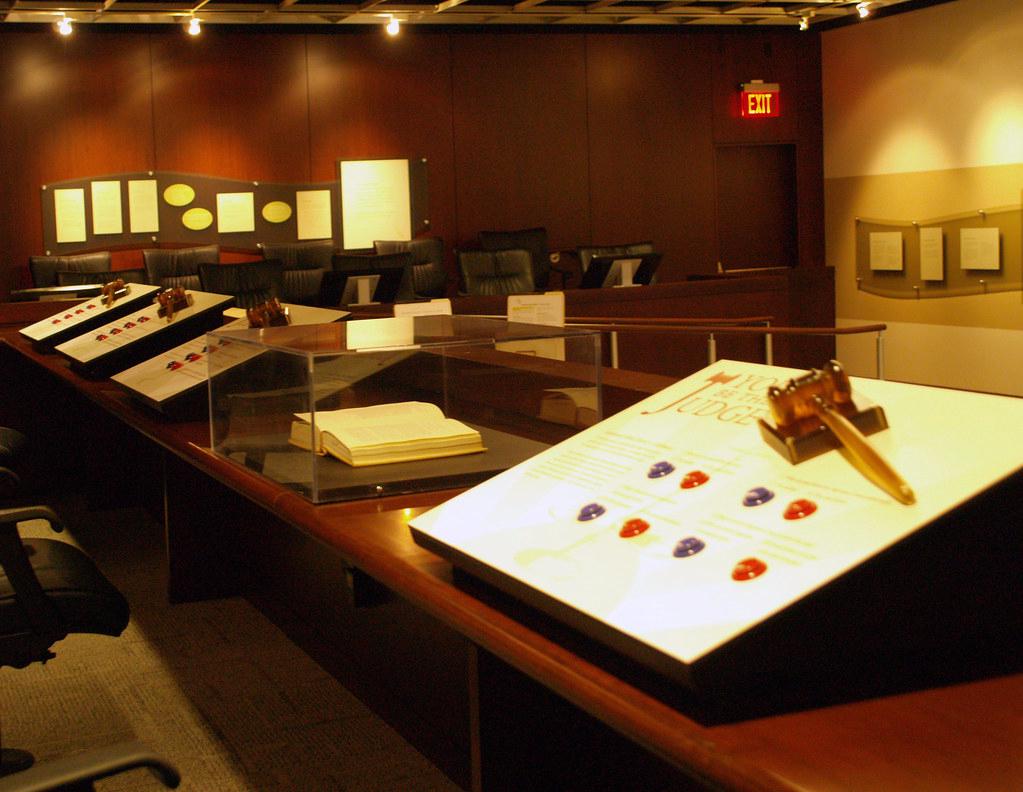
In high-stakes fields, knowing AI's decision-making process is crucial. By making AI's workings clearer, we tin physique spot and promote liable use, ensuring that these almighty tools are some effectual and transparent.
Writio: Your AI contented writer for websites and blogs. This station was crafted by Writio.
- IBM. Global AI Adoption Index. 2022.
- Adadi A, Berrada M. Peeking Inside the Black-Box: A Survey connected Explainable Artificial Intelligence (XAI). IEEE Access. 2018;6:52138-52160.
- Bibal A, Lognoul M, de Streel A, Frénay B. Legal Requirements connected Explainability successful Machine Learning. Artificial Intelligence and Law. 2021;29:149-169.
- Doshi-Velez F, Kim B. Towards A Rigorous Science of Interpretable Machine Learning. arXiv preprint arXiv:1702.08608. 2017.
- Miller T. Explanation successful Artificial Intelligence: Insights from the Social Sciences. Artificial Intelligence. 2019;267:1-38.