Current Climate Modeling Challenges
Climate models look important challenges successful accurately predicting aboriginal conditions. Some cardinal issues include:
- Systematic biases compared to observations
- Computational limitations restricting exemplary resolution
- Difficulty capturing small-scale processes similar unreality formation
High-resolution storm-resolving models (SRMs) connection improved accuracy but necessitate tremendous processing power. To code these limitations, researchers are exploring innovative approaches utilizing instrumentality learning, peculiarly heavy learning (DL).
DL shows committedness successful refining clime models by learning from SRM simulations. A cardinal absorption is integrating causal relationships into neural networks to amended exemplary show crossed varied clime conditions. These causally-informed neural networks purpose to discern existent causal drivers from specified correlations, perchance starring to much realistic and coherent clime simulations.
By focusing connected the main drivers of subgrid processes, these models question to trim overfitting and nutrient much reliable semipermanent forecasts. The attack involves cautiously identifying causal factors and incorporating them into neural web architectures optimized for clime prediction.
Role of High-Resolution Models
High-resolution storm-resolving models (SRMs) play a important relation successful advancing clime subject by capturing fine-scale atmospheric processes often missed by broader clime models. Some cardinal advantages of SRMs include:
- Resolving elaborate features similar tempest tracks and precipitation patterns
- Reducing biases contiguous successful coarser clime simulations
- Providing much close representations of important phenomena similar the Intertropical Convergence Zone
Despite their benefits, SRMs look important computational challenges. The tremendous processing powerfulness required limits their usage to abbreviated clip periods, typically conscionable a fewer months. This restricts their nonstop exertion for semipermanent clime projections.
However, SRMs stay invaluable tools for improving our knowing of Earth's clime systems. As exertion advances, the integration of high-resolution information into broader clime predictions is becoming progressively feasible. This advancement whitethorn yet alteration SRMs from specialized probe instruments into modular components of operational clime forecasting.
Machine Learning successful Climate Modeling
Machine learning, particularly heavy learning, is revolutionizing clime modeling by uncovering small-scale processes that accepted models conflict to capture. These AI techniques purpose to amended the simulation of captious subgrid-scale phenomena similar unreality enactment and convection.
ML algorithms survey high-resolution storm-resolving models to larn analyzable atmospheric processes. This cognition is past applied to refine accepted clime models, perchance bridging the spread betwixt fine-scale simulations and planetary predictions.
"ML methods for Earth strategy modeling is an progressive country of research. Particularly challenging to code are the mediocre practice of unseen climates and regimes (i.e., generalization capabilities) and hybrid modeling instabilities associated with the enactment betwixt the ML-based algorithm and the dynamical halfway of the big clime model."A cardinal absorption is the integration of causal relationships into ML models. This attack aims to marque predictions much unchangeable and reliable crossed assorted clime conditions. By distinguishing existent cause-effect pathways from spurious correlations, causally-informed neural networks whitethorn nutrient much close and physically accordant clime simulations.
As instrumentality learning advances successful clime science, it holds the imaginable to dramatically amended our quality to foretell Earth's analyzable clime systems. However, challenges stay successful ensuring these models generalize good to caller clime conditions and integrate seamlessly with existing carnal models.
Causally-Informed Neural Networks
Causally-informed neural networks correspond a cutting-edge attack to clime modeling, aiming to recognize the underlying reasons for clime phenomena. These networks effort to separate meaningful causes from coincidental correlations, perchance making models much robust to caller information and changing conditions.
Key features of causally-informed neural networks include:
- Focusing connected variables with genuine power implicit clime phenomena
- Identifying and prioritizing causal relationships
- Producing much reliable and interpretable clime predictions
By incorporating causal find methods, these networks tin prune spurious connections and absorption connected the astir important drivers of clime processes. This attack whitethorn pb to much physically accordant models that generalize amended to unseen clime regimes.
As these networks proceed to develop, they clasp the imaginable to heighten our knowing of atmospheric dynamics and amended the clarity and reliability of clime forecasts. However, challenges stay successful accurately identifying causal relationships successful complex, interconnected clime systems.
Applications and Case Studies
Deep learning models are demonstrating promising results successful predicting utmost upwind events with improved accuracy. Some notable applications include:
- Using convolutional neural networks to absorption connected determination clime changes
- Providing elaborate insights for section communities and policymakers
- Informing decision-making related to planetary agreements similar the Paris Protocol
These models connection granular views of imaginable somesthesia threshold breaches, helping to measure clime risks astatine determination scales. Such elaborate forecasts tin beryllium important for processing targeted adaptation and mitigation strategies.
Transfer learning techniques are being applied to determination clime models, perchance improving their realism by incorporating observational data. This attack allows models trained connected planetary information to beryllium fine-tuned for circumstantial regions, enhancing section predictions.
As AI-driven clime tools evolve, they whitethorn assistance displacement clime strategies from reactive to proactive approaches. By providing earlier and much close warnings of utmost events, these models could play a captious relation successful reducing climate-related risks and informing semipermanent planning.
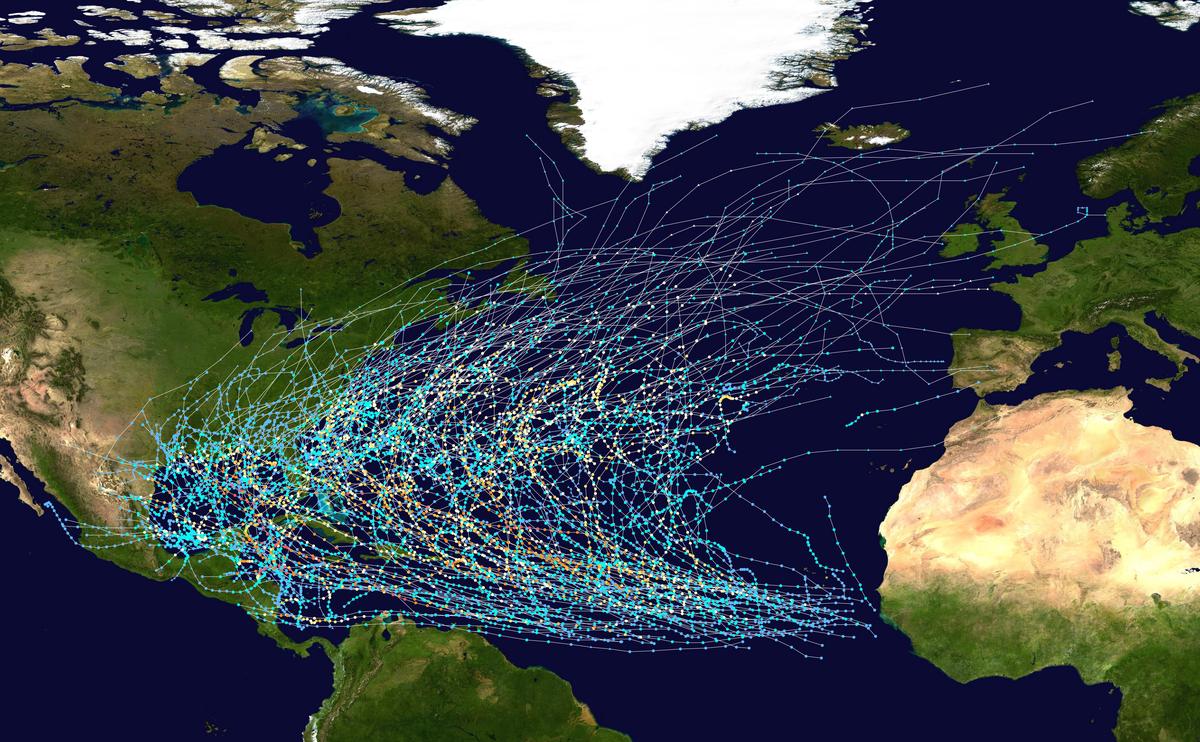
Writio: Your AI contented creator for websites and blogs. This station was written by Writio.
- Eyring V, Gillett NP, et al. Human power connected the clime system. In: Climate Change 2021: The Physical Science Basis. Cambridge University Press; 2021.
- Gentine P, Pritchard M, et al. Could instrumentality learning interruption the convection parameterization deadlock? Geophys Res Lett. 2018;45(11):5742-5751.
- Rasp S, Pritchard MS, Gentine P. Deep learning to correspond subgrid processes successful clime models. Proc Natl Acad Sci USA. 2018;115(39):9684-9689.
- Brenowitz ND, Beucler T, et al. Interpreting and stabilizing machine-learning parametrizations of convection. J Atmos Sci. 2020;77(12):4357-4375.
- Pearl J, Mackenzie D. The Book of Why: The New Science of Cause and Effect. Basic Books; 2018.